Does “spring forward” really make roads riskier? Arity driving behavior data may tell a different story Read article
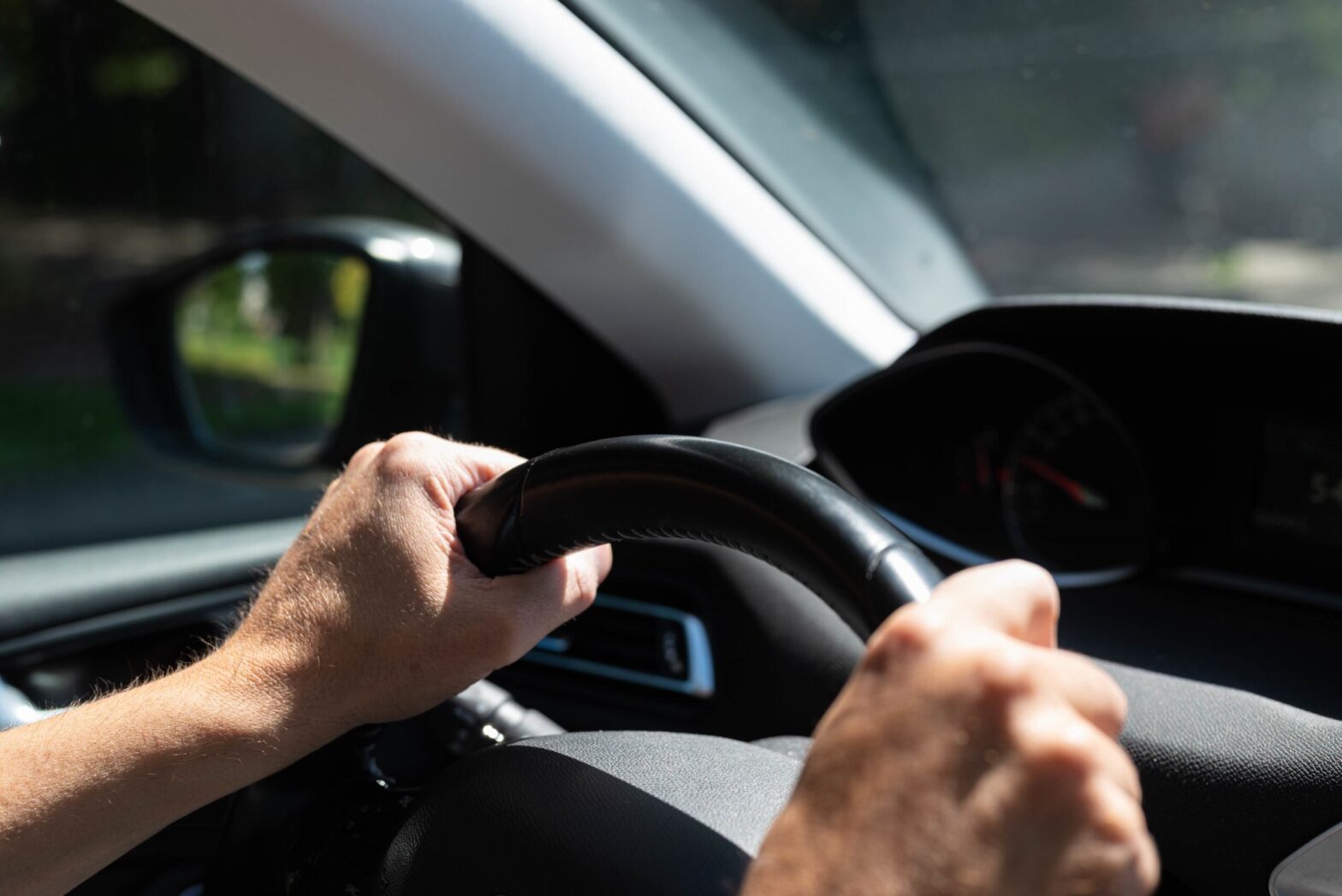
The rise of generative AI chatbots, such as ChatGPT (Generative Pre-trained Transformer), has captured the curiosity of millions since its inception last November.
However, the immense power of generative AI also raises concerns. Wired magazine described these chatbots as “silver-tongued agents of chaos.” Additionally, a team from Stanford University and the University of California, Berkeley noted in a research study the potential accuracy issue with newer iterations of GPT.
Amidst these uncertainties, most experts agree that generative AI will disrupt work as we know it today. A 2022 PwC survey found that 96% of the 1,000 survey respondents planned to use AI that year—from forecasting market conditions to supporting Internet of Things (IoT) initiatives. Moreover, in the 2023 report, “A new era of generative AI for everyone,” Accenture identified considerable potential for employing generative AI in the insurance industry, second only to the banking industry.
For insurers planning to leverage generative AI, recognizing the crucial role of data volume is imperative. Large language models (LLM) like generative AI heavily rely on unsupervised and semi-supervised machine learning algorithms, making the quantity of training data essential. Without sufficiently large data volume, the effectiveness of generative AI can suffer.
This is not unique to generative AI and applies to all predictive models. For instance, predictive models have been used extensively in the insurance industry. With the growing adoption of smartphones, connected vehicles, and other telematics devices around us, the auto insurance industry has access to more data than ever before.
The question is, how much data is enough for auto insurance telematics?
To explore the correlation between data volume and insurance risk prediction models based on driving behavior, data scientists and actuaries from Arity conducted two ground-breaking studies.
The first study was led by Ron Lettofsky, a lead data scientist and actuary. His team examined the financial impact of using too little data for training a driving risk prediction model. The main variable his team looked at was the amount of driving data in car years* coupled with the actual insurance loss claims needed to train a driving risk prediction model. His team was able to carry out this empirical study because Arity is connected to tens of millions of U.S. drivers (with consent) and hundreds of thousands of insurance claims data tied to telematics data.
What they found was illustrated in the accompanying chart. When an insufficient amount of data is used to train a driving risk prediction model, the impact on profitability not only can be negative (e.g., not charging risky drivers with sufficient premium to cover the potential losses from accidents), it can vary significantly as shown by the large bar on the left side of the chart. In contrast, when the driving risk prediction model is trained with the largest available actual driving data, the impact on profitability is not only positive but the variance is also much smaller as shown by the small bar on the right side of the chart.
The main takeaway is that by leveraging telematics scoring models built on large volumes of high-quality driving data, auto insurers can effectively charge all drivers more appropriate rates based on their driving behaviors. Applying risk-adjusted premiums to the safest and riskiest drivers enables auto insurers to improve the profitability of their book of business and remain competitive in the industry.
While it’s paramount to train a driving risk prediction model with actual driving data from as many drivers as possible while it’s being developed, it’s equally important to have continuous driving behavior data to achieve a high level of personalized prediction accuracy.
The second study, led by Patrick Peters, an actuary, delved into the impact of observation periods on the driving risk prediction accuracy on a single driver. His team discovered three crucial points:
Since its founding in 2016, Arity has collected more than 1 TRILLION miles of driving data. With insights on more than 30 million U.S. drivers, plus access to tens of thousands of actual loss claims, Arity can provide auto insurers with unmatched breadth and depth in driving behavior data.
A recently announced partnership with Connected Analytic Services, LLC (CAS) further enriches the scale of our dataset by incorporating data from connected Toyota and Lexus vehicles. This holistic view of driving behavior through continuously connected data from mobile devices AND connected vehicles can enable auto insurers to make highly accurate predictions of driving risk.
In conclusion, the growth of generative AI chatbots like ChatGPT is undeniably impressive. However, as with risk prediction models that lack sufficient data, the power of these technologies must be wielded with caution due to accuracy issues. For insurers seeking to adopt generative AI, recognizing the significance of data volume is paramount. In that regard, Arity’s vast and rich dataset provides unparalleled insights that can deliver positive and compelling business results for any auto insurer.
To learn more about how a partnership with Arity can make your insurance telematics solution more accurate and impactful, contact us today.
*One car year is equivalent to an insurance policy covering one car for a full year. Additionally, if three cars are insured for six months, this is equivalent to 1.5 car years.
** Written with contributions from Brian Faber, Patrick Peters, and Ron Lettofsky.