5 ways to “turbo-charge” auto insurance telematics Read article
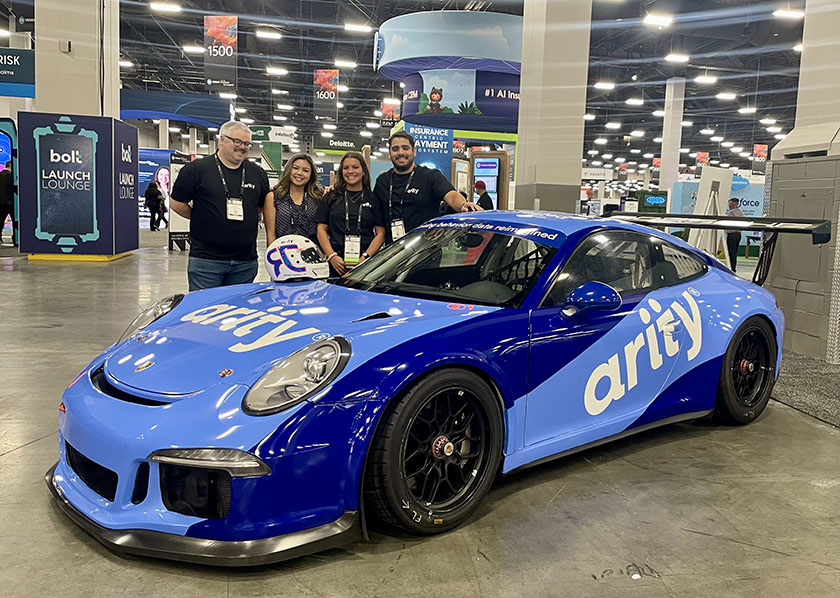
The transportation industry is evolving. Autonomous vehicles and Advanced Driver Assistance Systems (ADAS) promise lasting change. Unfortunately, an increase in distracted driving due to mobile phone use poses immediate challenges for the industry. And these short- and long-term changes require a modern telematics pricing model.
So how do we price insurance rates for this new normal? Insurance companies have long relied on demographic and credit data, but telematics data that measures drivers’ actual behaviors instead of reported behaviors, is the next step in understanding customers and predicting future losses.
Here at Arity, we use the following five criteria for accurate telematics pricing.
A telematics model should reflect actual insurance risk – after all, we’re in the risk business. We shouldn’t just model whether a crash event could take place. We must consider if the driving behaviors are actually causing insurance losses (frequency) and what the cost is to insurance (severity).
For example, our driving data looks at driving behaviors that have proven to result in costly incidents, like phone handling while driving, extreme speeding, and hard braking.
If driving behaviors are analyzed without adjustment for traditional rating factors, the correlation between these variables could be double-counted. For example, younger drivers tend to have more extreme driving behaviors compared with other cohorts. To some extent, traditional rating plans are already accounting for this. We need to be sure not to double count the effect of driving inexperience and isolate the impact of the actual driving behaviors.
Collision claims tend to be small but frequent, whereas bodily injury claims are infrequent but severe. Because the differences in resulting claims and severity varies significantly by coverage, modeling the impact of driving behaviors on insurance losses separately by coverage will enhance the predictive power of the overall rating plan. Arity has found that the variables we use change by coverage. For example, speed relative to the pace of the road might be more impactful for collision than comprehensive.
The truth is that hardware and mobile sensors collect different data. A hard fixed device may be able to read robust vehicle-specific data such as odometer and even noise level, while a mobile sensor can capture individual behaviors such as phone use and different modes of transportation. Even when the actual data elements are the same (e.g., GPS), the actual sensors’ quality and consistency vary. Normalization can be applied but in robust data sets it is proven that the best predictive power can only be obtained when the data is modeled by generalized sensor type. You’ll see new variables emerge and factors change.
The overwhelming majority of telematics programs deployed by insurance companies today include a consumer-facing experience and pricing adjustment based on actual driving behavior. Therefore, it’s critical that telematics pricing metrics are relevant to drivers. Customers need to understand the driving behaviors that affect their pricing so they can improve their driving habits and reduce their insurance costs.
Put together, these five components are more predictive of actual risk. Not only that, they benefit insurance customers. Mobility data helps us customize policies for drivers, avoid claims, and save money by encouraging good driving behavior. By providing transparent telematics insights, insurers can build customer trust and incentivize safer driving.
Using data for telematics pricing models prepares carriers for the “new normal.” And it positions them to transform the transportation industry as more accurate risk and predictive data emerges – it’s insurance 2.0.